Generative Adversarial Networks#
Summary#
Introduction and basic example
Deep Convolutional GANs
The GAN ecosystem
Introduction and basic example#
GAN in a nutshell#
First described in Generative Adversarial Nets by Goodfellow et al. (2014).
Simultaneously train two models:
One tries to generate realistic data.
The other tries to discriminate between real and generated data.
Each model is trained to best the other.
Training process#
Multiple iterations, each consisting of:
Phase 1
The generator creates images from random noise (typically a Gaussian distribution).
Generated images are mixed with real ones.
The discriminator is trained to distinguish fake vs. real images.
Phase 2
Another batch of images is produced by the generator.
The generator is trained so that the discriminator classifies these generated images as real.
Example: generating fashion images with a simple GAN#
(Heavily inspired by Chapter 17 of Hands-On Machine Learning by Aurélien Géron)
Environment setup#
import platform
print(f"Python version: {platform.python_version()}")
assert platform.python_version_tuple() >= ("3", "6")
import numpy as np
import matplotlib
import matplotlib.pyplot as plt
Python version: 3.7.5
# Setup plots
%matplotlib inline
plt.rcParams["figure.figsize"] = 10, 8
%config InlineBackend.figure_format = 'retina'
import tensorflow as tf
print(f"TensorFlow version: {tf.__version__}")
print(f"Keras version: {tf.keras.__version__}")
from tensorflow.keras import Model
from tensorflow.keras.models import Sequential
from tensorflow.keras.layers import (
Dense,
Flatten,
Reshape,
BatchNormalization,
Conv2D,
Conv2DTranspose,
LeakyReLU,
Dropout
)
from tensorflow.keras.datasets import fashion_mnist
TensorFlow version: 2.3.1
Keras version: 2.4.0
Data loading#
# Load training inputs from the Fashion-MNIST dataset
(train_images, _), (_, _) = fashion_mnist.load_data()
# Change pixel values from (0, 255) to (0, 1)
x_train = train_images.astype("float32") / 255
print(f"x_train: {x_train.shape}")
x_train: (60000, 28, 28)
Show code cell source
def plot_multiple_images(images, n_cols=None):
n_cols = n_cols or len(images)
n_rows = (len(images) - 1) // n_cols + 1
if images.shape[-1] == 1:
images = np.squeeze(images, axis=-1)
plt.figure(figsize=(n_cols * 1.5, n_rows * 1.5))
for index, image in enumerate(images):
plt.subplot(n_rows, n_cols, index + 1)
plt.imshow(image, cmap="binary")
plt.axis("off")
Model definition#
# Input dimensionality for generator
codings_size = 30
generator = Sequential(
[
Dense(100, activation="selu", input_shape=(codings_size,)),
Dense(150, activation="selu"),
Dense(28 * 28, activation="sigmoid"),
Reshape((28, 28)),
],
name="generator"
)
discriminator = Sequential(
[
Flatten(input_shape=(28, 28)),
Dense(150, activation="selu"),
Dense(100, activation="selu"),
Dense(1, activation="sigmoid"),
],
name="discriminator"
)
gan = Sequential([generator, discriminator])
# Print GAN model summary
gan.summary()
Model: "sequential"
_________________________________________________________________
Layer (type) Output Shape Param #
=================================================================
generator (Sequential) (None, 28, 28) 136634
_________________________________________________________________
discriminator (Sequential) (None, 1) 132951
=================================================================
Total params: 269,585
Trainable params: 269,585
Non-trainable params: 0
_________________________________________________________________
Model training#
# The generator is trained through the GAN model: no need to compile it
discriminator.compile(loss="binary_crossentropy", optimizer="rmsprop")
# The trainable attribute is taken into account only when compiling a model
# Discriminator weights will be updated only when it will be trained on its own
# They will be frozen when the whole GAN model will be trained
discriminator.trainable = False
gan.compile(loss="binary_crossentropy", optimizer="rmsprop")
def train_gan(gan, dataset, batch_size, codings_size, n_epochs=50):
generator, discriminator = gan.layers
for epoch in range(n_epochs):
print(f"Epoch [{epoch+1}/{n_epochs}]...")
for x_batch in dataset:
# Phase 1 - training the discriminator
noise = tf.random.normal(shape=(batch_size, codings_size))
generated_images = generator(noise)
# Gather an equal number of generated (y=0) and real (y=1) images
x_discr = tf.concat([generated_images, x_batch], axis=0)
y_discr = tf.constant([[0.0]] * batch_size + [[1.0]] * batch_size)
# https://stackoverflow.com/a/49100617
discriminator.train_on_batch(x_discr, y_discr)
# Phase 2 - training the generator
noise = tf.random.normal(shape=(batch_size, codings_size))
# Generated images should be labeled "real" by the discriminator
y_gen = tf.constant([[1.0]] * batch_size)
# Update only the generator weights (see above)
gan.train_on_batch(noise, y_gen)
print("Training complete!")
batch_size = 32
# Load images in batches
dataset = tf.data.Dataset.from_tensor_slices(x_train).shuffle(1000)
dataset = dataset.batch(batch_size, drop_remainder=True).prefetch(1)
# Train the GAN model
train_gan(gan, dataset, batch_size, codings_size, n_epochs=1)
Epoch [1/1]...
Training complete!
Generating new images#
noise = tf.random.normal(shape=(batch_size, codings_size))
generated_images = generator(noise)
plot_multiple_images(generated_images, 8)
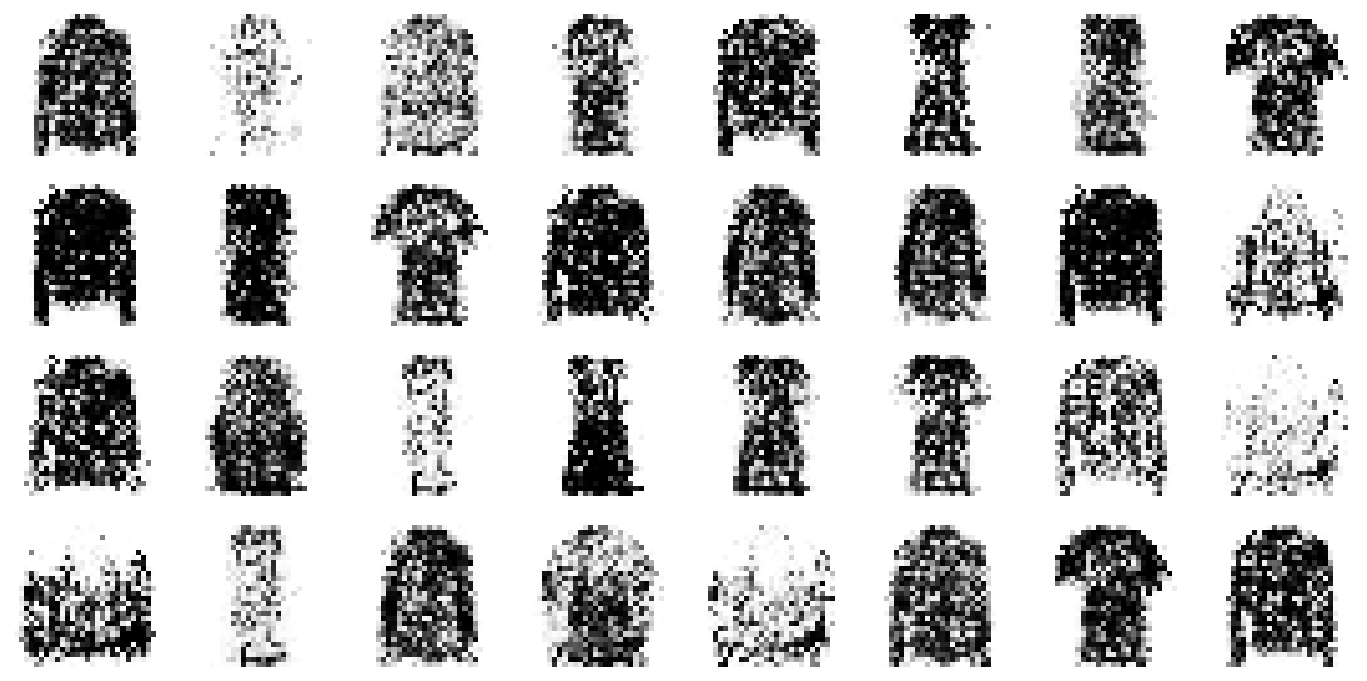
GAN specificities and gotchas#
A GAN is a dynamic system that evolves at each training step.
Interestingly, the generator never sees images froms the training set directly: all it gets are the gradients coming back from the discriminator.
Training can be tricky with several pitfalls: noisy generated data, vanishing gradients, lack of diversity in generated data, domination of one side…
GAN convergence theory is an active area of research.
Deep Convolutional GANs#
Architecture#
A few months after the original GAN paper came out, a team succeeded in building GANs based on deeper convolutional models, or DCGANs (paper).
General rules for implemeting DCGANs are:
Replace pooling layers with strided convolutions (in the discriminator) and transposed convolutions (in the generator).
Add batch normalization.
Use ReLU in the generator except for the output layer, which should use tanh.
Use leaky ReLU in the discriminator.
The leaky ReLU activation function#
Leaky version of a Rectified Linear Unit. It allows a small gradient when the unit is not active to prevent the “dying ReLU” problem (neurons always outputting zero).
Example: generating fashion images with a DCGAN#
(Heavily inspired by Chapter 17 of Hands-On Machine Learning by Aurélien Géron)
Generator definition#
# Input dimensionality for generator
codings_size = 100
dcgan_generator = Sequential(
[
Dense(7 * 7 * 128, input_shape=(codings_size,)),
Reshape((7, 7, 128)),
BatchNormalization(),
Conv2DTranspose(
64, kernel_size=5, strides=2, padding="same", activation="selu"
),
BatchNormalization(),
Conv2DTranspose(1, kernel_size=5, strides=2, padding="same", activation="tanh"),
],
name="generator",
)
dcgan_generator.summary()
Model: "generator"
_________________________________________________________________
Layer (type) Output Shape Param #
=================================================================
dense_23 (Dense) (None, 6272) 633472
_________________________________________________________________
reshape_12 (Reshape) (None, 7, 7, 128) 0
_________________________________________________________________
batch_normalization_20 (Batc (None, 7, 7, 128) 512
_________________________________________________________________
conv2d_transpose_20 (Conv2DT (None, 14, 14, 64) 204864
_________________________________________________________________
batch_normalization_21 (Batc (None, 14, 14, 64) 256
_________________________________________________________________
conv2d_transpose_21 (Conv2DT (None, 28, 28, 1) 1601
=================================================================
Total params: 840,705
Trainable params: 840,321
Non-trainable params: 384
_________________________________________________________________
Discriminator definition#
dcgan_discriminator = Sequential(
[
Conv2D(
64,
kernel_size=5,
strides=2,
padding="same",
activation=LeakyReLU(0.2),
input_shape=(28, 28, 1),
),
Dropout(0.4),
Conv2D(
128, kernel_size=5, strides=2, padding="same", activation=LeakyReLU(0.2)
),
Dropout(0.4),
Flatten(),
Dense(1, activation="sigmoid"),
],
name="discriminator",
)
dcgan_discriminator.summary()
Model: "discriminator"
_________________________________________________________________
Layer (type) Output Shape Param #
=================================================================
conv2d_11 (Conv2D) (None, 14, 14, 64) 1664
_________________________________________________________________
dropout_10 (Dropout) (None, 14, 14, 64) 0
_________________________________________________________________
conv2d_12 (Conv2D) (None, 7, 7, 128) 204928
_________________________________________________________________
dropout_11 (Dropout) (None, 7, 7, 128) 0
_________________________________________________________________
flatten_6 (Flatten) (None, 6272) 0
_________________________________________________________________
dense_24 (Dense) (None, 1) 6273
=================================================================
Total params: 212,865
Trainable params: 212,865
Non-trainable params: 0
_________________________________________________________________
Model definition#
dcgan = Sequential([dcgan_generator, dcgan_discriminator])
dcgan.summary()
Model: "sequential_7"
_________________________________________________________________
Layer (type) Output Shape Param #
=================================================================
generator (Sequential) (None, 28, 28, 1) 840705
_________________________________________________________________
discriminator (Sequential) (None, 1) 212865
=================================================================
Total params: 1,053,570
Trainable params: 1,053,186
Non-trainable params: 384
_________________________________________________________________
Model training#
# The generator is trained through the GAN model: no need to compile it
dcgan_discriminator.compile(loss="binary_crossentropy", optimizer="rmsprop")
# The trainable attribute is taken into account only when compiling a model
# Discriminator weights will be updated only when it will be trained on its own
# They will be frozen when the whole GAN model will be trained
dcgan_discriminator.trainable = False
dcgan.compile(loss="binary_crossentropy", optimizer="rmsprop")
# Reshape and rescale input into a 4D tensor with values between -1 and 1
# Needed because tanh outputs are in this range
x_train_dcgan = x_train.reshape(-1, 28, 28, 1) * 2. - 1.
print(f"x_train_dcgan: {x_train_dcgan.shape}")
x_train_dcgan: (60000, 28, 28, 1)
batch_size = 32
# Load images in batches
dataset = tf.data.Dataset.from_tensor_slices(x_train_dcgan)
dataset = dataset.shuffle(1000)
dataset = dataset.batch(batch_size, drop_remainder=True).prefetch(1)
# Train the DCGAN model
train_gan(dcgan, dataset, batch_size, codings_size, n_epochs=5)
Epoch [1/5]...
Epoch [2/5]...
Epoch [3/5]...
Epoch [4/5]...
Epoch [5/5]...
Training complete!
Generating new images#
noise = tf.random.normal(shape=(batch_size, codings_size))
generated_images = dcgan_generator(noise)
plot_multiple_images(generated_images, 8)
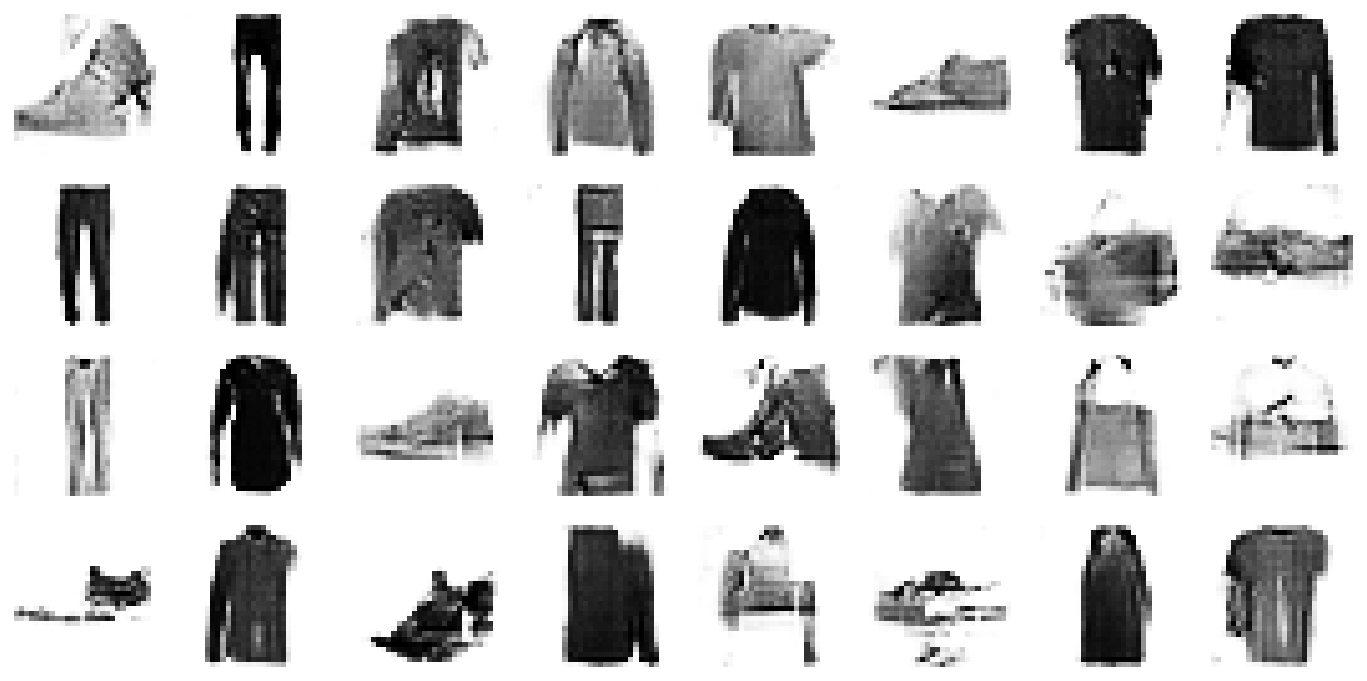
The GAN ecosystem#
A GAN landscape#
GAN progress on face generation#
GAN use cases: not just images!#
Writing a novel “in the style of an author”.
Generating realistic passwords for hackers.
Generating videos (example).
…